Congratulations to the Challenge Winners!
Congratulations to the Better Meter Stick for Differential Privacy Challenge Winners!
Thank you to all the contestants and everyone who made this contest a success. The judges were impressed and excited by many of the innovative ideas in the entries.
Judges reviewed and determined 4 entries to be eligible for the Technical Merit prizes and the Public Choice prizes. Please see below to read about the winning teams and entries.
Selected metrics developed by the winners may be used to evaluate differential privacy algorithms submitted to sprint 3 of the Differential Privacy Temporal Map Contest.
First Prize: $5,000
One First Prize award was granted.
Submission Name: MGD: A Utility Metric for Private Data Publication
Team member(s): Ninghui Li, Trung Đặng Đoàn Đức, Zitao Li, Tianhao Wang
Location: West Lafayette, IN; Vietnam; China
Affiliation: Purdue University
Who is your team and what do you do professionally?
We are a research group from Purdue University working on differential privacy. Our research group has been conducting research on data privacy for about 15 years, with a focus on differential privacy for the most recent decade. Our group has developed state-of-the-art algorithms for several tasks under the constraint of satisfying Differential Privacy and Local Differential Privacy.
What motivated you to compete in this challenge?
We have expertise in differential privacy. We also participated in earlier competitions held by NIST and got very positive results. We believe this is a good opportunity to think more about real world problems and explore the designs of metrics for evaluating quality of private dataset.
High level summary of approach
We propose MarGinal Difference (MGD), a utility metric for private data publication. MGDassigns a difference score between the synthesized dataset and the ground truth dataset. The high level idea behind MGD is to measure the differences between many pairs marginal tables, each pair having one computed from the two datasets. For measuring the difference between a pair of marginal tables, we introduce Approximate Earth Mover Cost, which considers both semantic meanings of attribute values and the noisy nature of the synthesized dataset.
Second Prize: $3,000
Two Second Prize awards were granted.
Submission Name: Practical DP Metrics
Team Member(s): Bolin Ding, Xiaokui Xiao, Ergute Bao, Jianxin Wei, Kuntai Cai
Location: China
Affiliations: Alibaba Group and the National University of SIngapore
- Who is your team and what do you do professionally?
We are a group of researchers interested in differential privacy.
2. What motivated you to compete in this challenge?
To apply our research on differential privacy in a practical setting.
3. High level summary of approach
We introduce four additional metrics for the temporal data challenge, evaluating the Jaccard distance, heavy hitters, and horizontal and vertical correlations. We motivate these metrics with real-world applications. We show that these additional metrics can complement the JSD metric currently used in the challenge, to provide more comprehensive evaluation.
Submission Title: Confusion Matrix Metric
Team Member(s): Sowmya Srinivasan
Location: Alameda, California
Who are you and what do you do professionally?
My name is Sowmya and I am a Data Analyst/Scientist with a background in Astrophysics. At the moment, I am employed by bettercapital.us as a Data Intern but am seeking a full-time position as a Data Analyst/Data Scientist. I have a certificate from a Data Analytics and Visualization bootcamp and I have a lot of experience working with large datasets thanks to the bootcamp as well as my Astrophysics background. When I am not working on projects my hobbies include reading and cooking.
What motivated you to compete in this challenge?
I was looking into expanding my understanding of data science/analytics and decided to browse on challenge.gov to see if there were any projects I could apply my current knowledge to and found this challenge. I was immediately interested in the motivation as I am highly intrigued by privacy methods and how to work with them. In addition, I have been looking into learning more about metrics so that was also appealing.
High level summary of approach
The confusion matrix metric is essentially a more complex version of the pie chart metric provided for the challenge. The pie chart metric consists of three components: one that evaluates the Jensen-Shannon distance between the privatized and ground truth data, one that penalizes false positives in privatized data, and one that penalizes large total differences between the privatized and ground truth data. The confusion matrix metric adds two elements onto this metric: an element that penalizes for large shifts in values within a record as well as an element that measures the differences in time-series pattern between the ground truth and the privatized dataset. The first element is evaluated through binning values and adding on a penalty if the values change bins after privatization. The second element uses the r-squared value between the two over a chosen time-segment.
The confusion matrix representation shows the percent of false positives and false negatives in a privatized record. Its purpose is to provide an easy way to view the utility of a particular record or the entire dataset.
Another visualization that may be insightful is the bar chart depicting the component that penalizes for change in rank. This is a way to show how the values are separated into bins and how those bin sizes compare with those of the ground truth dataset.
Third Prize: $2,000
One Third Prize award was granted.
Submission Title: Bounding Utility Loss via Classifiers
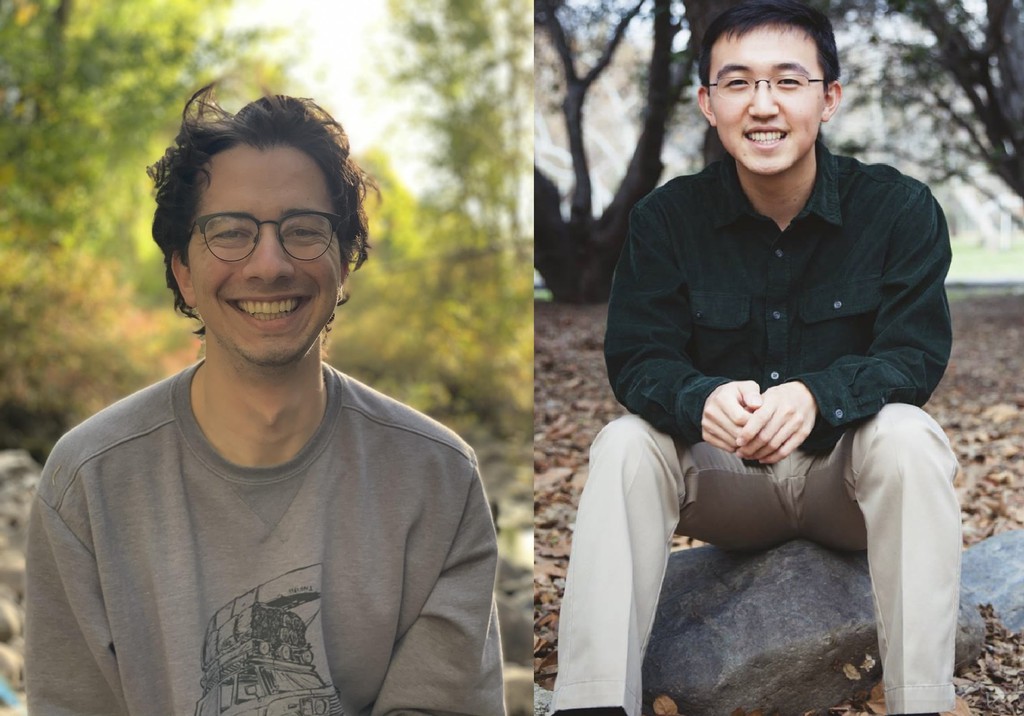
Team Member(s): Leo Hentschker and Kevin Lee
Location: Montclair, New Jersey and Irvine, California
Who are you and what do you do professionally?
Leo Hentschker: After his freshman year at Harvard, Leo dropped out to help found Quorum Analytics, a legislative affairs software startup focused on building a "Google for Congress." After helping to scale the company and returning to school, he graduated in three years with honors with a degree in mathematics. He is now the CTO at Column, an early stage startup focused on improving the utility of public interest information.
Kevin Lee: Kevin is a PhD student in economics at the University of Chicago, Booth School of Business, studying the design of platform markets. He is interested in fixing market failures in digital advertising and how reputation systems shape incentives for product quality. In the past he won 2nd place in the Intel Science Talent Search and graduated with a degree in applied math from Harvard.
What motivated you to compete in this challenge?
At Column, Leo has seen first hand how the lack of transparency hurts local communities across the country, and how improper applications of privacy can leave individuals vulnerable. Formal guarantees around utility of privatized datasets would meaningfully improve Column's ability to disclose public interest information in a way that is useful to the public and protects individual privacy.
Kevin believes that tensions between transparency and privacy create inefficient market structures that harm consumers and companies. Principled application of differential privacy has the potential to resolve this tradeoff.
Summary of approach
If a classifier can easily distinguish between privatized and ground truth data, the datasets are fundamentally different, and the privatized data should not be used for downstream analysis. Conversely, if a classifier cannot distinguish them, we should feel comfortable using the privatized data going forward. In the latter case, we prove that any classifier from the same function family will have essentially the same loss on your private and ground truth data.
We define a normalized version of this maximum difference in loss as the separability and provide an algorithm for computing it empirically.
People's Choice Prize: $1,000
One People's Choice award was granted.
Submission Name: Confusion Matrix Metric
Team Member(s): Sowmya Srinivasan